Bayesian Face Revisited: A Joint Formulation
Abstract In this paper, we revisit the classical Bayesian face recognition method by Baback Moghaddam et al. and propose a new joint formulation. The classical Bayesian method models the appearance difference between two faces. We observe that this "difference" formulation may reduce the separability between classes. Instead, we model two faces jointly with an appropriate prior on the face representation. Our joint formulation leads to an EM-like model learning at the training time and an efficient, closed-formed computation at the test time. On extensive experimental evaluations, our method is superior to the classical Bayesian face and many other supervised approaches. Our method achieved 92.4% test accuracy on the challenging Labeled Face in Wild (LFW) dataset. Comparing with current best commercial system, we reduced the error rate by 10%.
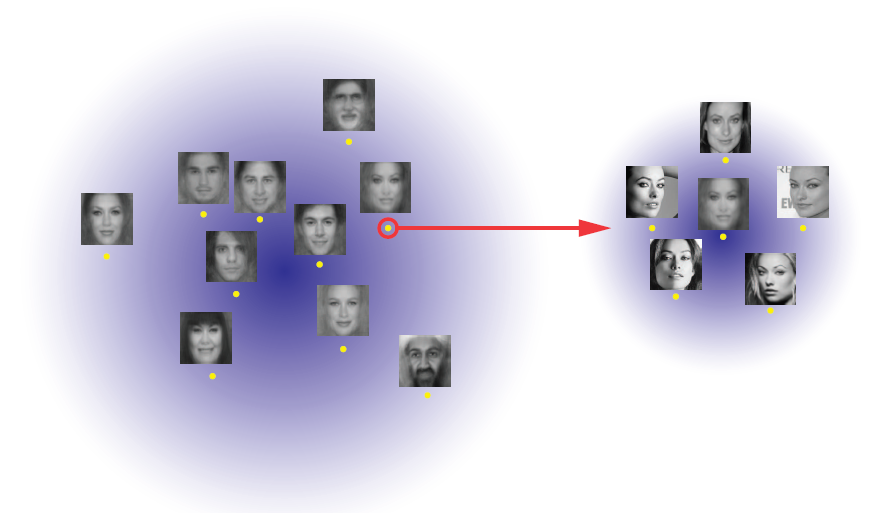
[PDF] [Supplemental] [WDRef database]